Strategies for Accurate Unstructured Data Discovery
In today’s era of big data, accurately discovering and managing unstructured data is critical. This type of data, encompassing formats such as emails, documents, and multimedia, constitutes upwards of 80-90% of all digital data. Although rich in information, unstructured data lacks standardization, presenting unique challenges in its management and governance.
Today’s post explores effective strategies and best practices for accurately discovering unstructured data, a foundational component in ensuring data security, compliance, and informed decision-making.
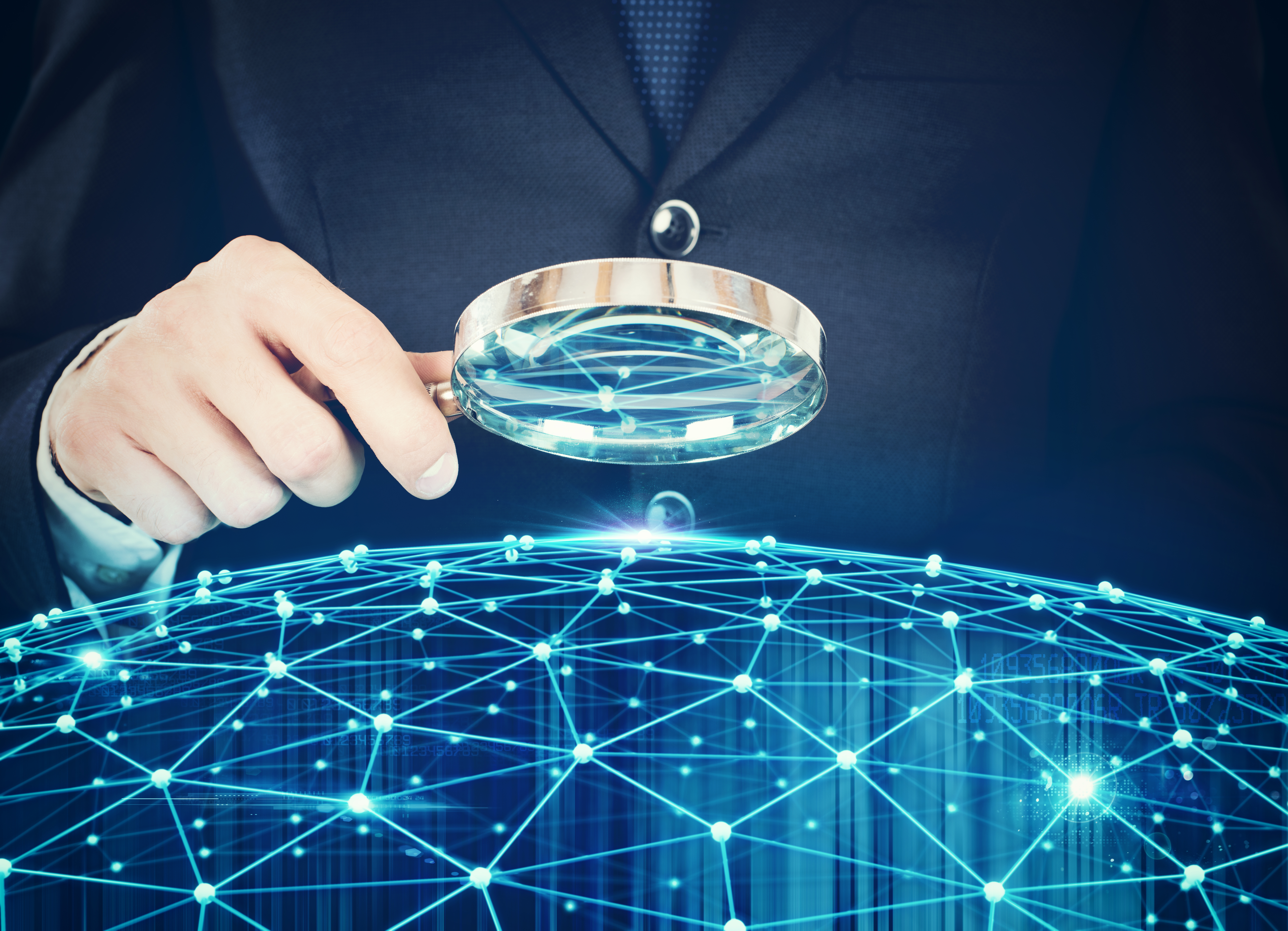
The Importance of Accuracy in Unstructured Data Discovery
Accurate data discovery, especially in the context of unstructured data, involves identifying and categorizing data that lacks a standard format or structure. This process is vital for understanding the context, relevance, and sensitivity of the data. Accurate discovery is essential for maintaining robust data security, privacy, and compliance.
Inadequate discovery leaves sensitive data exposed, increasing the risk of breaches. The average cost of a data breach is approximately $4.35 million (IBM, 2023), highlighting the significant financial impact of data vulnerabilities. Furthermore, with the enactment of comprehensive consumer data protection in 12 U.S. states, in addition to the EU’s GDPR and China’s PIPL, accurate data discovery has become increasingly critical for compliance. Beyond compliance, accurate data discovery is instrumental in efficient data management and informed decision-making, thereby influencing overall business operations.
Why Accuracy is Critical in Data Discovery
Accuracy in sensitive data discovery is fundamental to ensuring robust data security, maintaining privacy, adhering to compliance requirements, and implementing effective data governance. It transcends mere compliance to become a broader business imperative, impacting an organization’s risk profile, operational effectiveness, and overall reputation including:
- Compliance with Data Protection Regulations
- Regulatory Requirements: Laws like GDPR, CPRA, and HIPAA impose strict rules on handling sensitive information, including unstructured data.
- Audit and Reporting: Accurate data discovery is essential for compliance auditing and reporting, with inaccuracies potentially leading to failed audits and legal issues.
- Protection Against Data Breaches and Security Incidents
- Vulnerability Identification: Precise discovery is key to identifying where sensitive unstructured data resides, crucial for applying appropriate security measures.
- Risk Management: Accurate identification of sensitive data allows organizations to better assess risk exposure and develop targeted security strategies.
- Incident Response: Knowing exactly what data has been compromised is vital for an effective breach response and mitigation strategy.
- Maintaining Consumer Trust and Brand Reputation
- Consumer Confidence: Protecting sensitive information through accurate discovery is essential to maintaining consumer trust.
- Brand Integrity: Data breaches or compliance failures stemming from inaccurate unstructured data discovery can severely damage an organization’s reputation.
- Operational Efficiency and Decision Making
- Data Utilization: Accurate discovery ensures sensitive data is correctly identified and utilized, enhancing operational efficiency.
- Informed Decision Making: Accurate categorization of sensitive data aids in making decisions based on complete and reliable datasets.
- Ethical Responsibilities and Privacy Concerns
- Ethical Use of Data: Accurate discovery is fundamental for the ethical handling of sensitive data, preventing misuse, particularly in AI training data sets.
- Privacy Protection: Protecting individuals’ privacy is an ethical obligation; inaccurate discovery can lead to privacy violations.
- Facilitating Data Accessibility and Portability
- Data Subject Rights: CPRA and similar regulations grant individuals rights over their data. Accurate discovery is essential to fulfill these rights effectively.
- Data Portability: Efficient data portability, enabling individuals to reuse their personal data across services, relies on accurate unstructured data discovery.
- Minimizing Data Redundancy and Storage Costs
- Reduced Redundancy: Identifying redundant copies of sensitive data, which can be securely deleted, helps reduce storage costs.
- Optimized Storage Management: Accurate identification of sensitive data aids in optimizing data storage and management strategies.
Consequences of Inaccurate Data Discovery
The consequences of inaccurate unstructured data discovery can be far-reaching, affecting not just the operational aspects of an organization but also its legal standing and reputation. It can lead to increased vulnerability to breaches, ineffective data monitoring, compromised data integrity, privacy violations, non-compliance with regulations, financial penalties, auditing challenges, impaired decision-making, data lifecycle management issues, and resource misallocation.
Inaccurate unstructured data discovery can result in:
Data Security
- Increased Vulnerability to Breaches: Inaccurate data discovery can leave sensitive or critical data unidentified and unprotected, making it more susceptible to breaches. Without a clear understanding of where sensitive data resides, security measures cannot be effectively applied.
- Ineffective Data Monitoring: If data discovery is inaccurate, monitoring systems may fail to flag unauthorized access or unusual activity, as they are not tuned to the correct data sets.
- Compromised Data Integrity: Inaccurate data discovery can lead to data corruption or loss, as backup and recovery processes may not encompass all critical unstructured data.
Privacy
- Privacy Violations: Inaccurate discovery can result in the mishandling of personal information, leading to privacy violations.
- Inadequate Data Anonymization: Overlooked data sets might not be properly anonymized or encrypted, leading to potential exposure of private information.
- Loss of Consumer Trust: Privacy breaches, often stemming from poor data management, can significantly erode trust between the organization and its customers or users.
Compliance
- Non-Compliance with Regulations: Many industries are governed by strict data protection regulations like HIPAA, FERPA, and PCI-DSS. Inaccurate unstructured data discovery can lead to non-compliance, as organizations might fail to adequately protect or report on the handling of sensitive data.
- Financial Penalties: Non-compliance can result in substantial fines. For instance, GDPR violations can lead to fines of up to 4% of annual global turnover or €20 million.
- Auditing Challenges: Inaccurate data discovery complicates compliance auditing processes, potentially leading to failed audits and the associated legal and financial repercussions.
Governance
- Impaired Decision Making: Data governance is crucial for informed decision-making. Inaccurate data discovery can lead to decisions based on incomplete or incorrect data, affecting business strategies and operations.
- Data Lifecycle Management Issues: Effective data governance requires understanding the lifecycle of data. Inaccurate discovery can result in poor data lifecycle management, leading to data being stored longer than necessary or disposed of prematurely.
- Resource Misallocation: Inaccurate data discovery can lead to misallocation of resources, as efforts may be focused on less critical data, while key data sets are neglected.
Challenges in Unstructured Data Discovery
Achieving accuracy in unstructured data discovery is fraught with challenges that are both technical and organizational in nature. The top issues include the sheer volume of data, the variety of data formats, and the rapid rate at which data is generated and modified. Understanding these challenges is the first step towards developing effective strategies to overcome them.
Key hurdles in accurate data discovery include:
- Volume and Complexity: The sheer volume of unstructured data, growing exponentially, makes it difficult to manage and analyze effectively. This data often exists in diverse formats – from emails and documents to social media content and multimedia – further adding to the complexity.
- Accuracy and Efficiency: Ensuring high accuracy in data discovery is a significant challenge, particularly when dealing with vast amounts of unstructured data. Tools must not only be precise but also efficient to handle the data load without causing delays in processing.
- Complexity of Data Storage: Data stored across multiple platforms adds complexity to discovery.
- Integration with Existing Systems: Many organizations struggle to integrate new data discovery tools with their existing IT infrastructure. This can lead to siloed data and inefficiencies in the discovery process.
- Scalability: As organizations grow, their data discovery solutions must scale accordingly. Scalability issues can lead to incomplete data discovery and management challenges.
- Data Sensitivity Identification: Accurately identifying and classifying sensitive data is complex and is not as straightforward to identify and manage compared to structured data.
- Evolving Compliance Requirements: Adapting the discovery and classification process to changing regulations is a continuous struggle.
- Cross-Departmental Coordination: Effective unstructured data discovery requires collaboration across various departments, including IT, legal, and compliance. Lack of coordination can lead to gaps in data discovery and management.
- Resource Allocation: Allocating adequate resources – both in terms of budget and personnel – for unstructured data discovery is often a challenge, especially for organizations where data management is not the core business function.
- Change Management: Implementing new processes and tools for data discovery often requires significant change management, as it involves altering existing workflows and training employees.
Technical Limitations of Today’s Data Discovery Solutions
Today’s data discovery solutions face numerous technical challenges, particularly when dealing with the complexity and variability of unstructured sensitive data. These include limited contextual understanding, inadequate classification algorithms, challenges with data in varied formats, scalability issues, dependency on pre-defined rules and patterns, integration and compatibility challenges, language and semantic analysis limitations, and more.
Here are the key technical limitations:
- Limited Contextual Understanding: Many data discovery tools struggle with understanding the context in which data exists, especially in unstructured formats. Contextual nuances, such as the significance of certain phrases or words in specific documents, can be challenging to interpret. This limitation can lead to both false positives and false negatives in identifying sensitive data.
- Inadequate Classification Algorithms: The algorithms used for classifying data, particularly unstructured data, may not be sophisticated enough to accurately distinguish between sensitive and non-sensitive information. Misclassification can result in either overprotecting data (leading to inefficiencies) or under protecting genuinely sensitive information (leading to security risks).
- Challenges with Data in Varied Formats: Unstructured data comes in a wide variety of formats, and many discovery tools are not equipped to handle this diversity effectively. Important sensitive data embedded in less common formats or complex file types might be overlooked.
- Scalability Issues: As the volume of data grows, some tools struggle to scale effectively, leading to decreased performance and accuracy. This can result in incomplete data discovery, especially in large or rapidly growing data environments.
- Dependency on Pre-Defined Rules and Patterns: Many tools rely heavily on pre-defined rules and patterns to identify sensitive data. This approach can be limiting, as it may not capture all types of sensitive information. Novel or unexpected types of sensitive data may go undetected.
- Integration and Compatibility Challenges: Integrating data discovery tools with existing systems and ensuring compatibility across different platforms and data sources can be challenging. Poor integration can lead to gaps in data discovery and protection.
- Language and Semantic Analysis Limitations: Tools may have limited capability in understanding different languages and semantics, which is crucial for global organizations dealing with multilingual data. Sensitive data in non-primary languages or with complex semantics may be missed.
- Real-Time Processing Constraints: The ability to process and analyze data in real-time is still limited in many tools, which is crucial for timely discovery of sensitive data. Delays in discovering sensitive data can lead to prolonged exposure and increased risk.
- AI and Machine Learning Shortcomings: While AI and ML have the potential to significantly alter the data discovery landscape, current implementations may have limitations in accuracy, bias, and learning capacity. AI-driven tools might incorrectly categorize data or miss subtle indications of sensitivity.
- User Error and Training Requirements: The effectiveness of data discovery tools can be hampered by user error or lack of adequate training. Even the most advanced tools can yield poor results if not used correctly.
Practical Solutions and Strategies for Effective Unstructured Data Discovery
In the complex world of data management, particularly with the ever-growing volume of unstructured data, organizations face significant challenges in accurately discovering and managing this information asset. However, there are actionable strategies and best practices that can streamline this process, ensuring both efficiency and compliance.
Effective strategies for unstructured data discovery involve a combination of advanced technology and robust processes. Implementing tools that employ AI and machine learning can significantly enhance the accuracy of unstructured data discovery. Regular audits and updates ensure that the discovery process remains effective over time. Additionally, educating employees about the importance of data categorization and the use of discovery tools can reduce the risk of human error.
Here are some practical tips for effective and accurate unstructured data discovery:
- Leverage Advanced Discovery Tools
- Implement AI-Powered Solutions: Utilize AI and machine learning tools that can intelligently categorize and manage unstructured data. These technologies can recognize patterns, classify data, and even predict where sensitive information is likely to be found.
- Automate Where Possible: Automation can significantly reduce the manual workload involved in data discovery. Automated tools can continuously scan and analyze data, ensuring nothing is overlooked.
- Regular Data Audits
- Conduct Periodic Reviews: Regularly audit your data to ensure that all sensitive information is accurately identified and properly managed. This also helps in keeping up with any changes in data privacy laws and regulations.
- Involve Cross-Functional Teams: Include various departments in these audits, such as IT, legal, and compliance, to ensure a comprehensive approach.
- Data Mapping and Classification
- Develop a Data Map: Create a map of where different types of data are stored within your organization. This helps in understanding the flow of information and identifying potential risk areas.
- Classify Data Effectively: Establish clear criteria for what constitutes sensitive, confidential, or public data. Proper classification is key to effective data management and security.
4. Employee Training and Awareness
- Educate Your Workforce: Regular training sessions for employees on the importance of data privacy and the role they play in data discovery can be invaluable.
- Promote a Culture of Data Responsibility: Encourage a workplace culture where data protection is a shared responsibility.
5. Stay Updated with Compliance Requirements
- Monitor Regulatory Changes: Keep abreast of changes in data protection laws and ensure your data discovery practices comply with these regulations.
- Implement Compliance-Focused Processes: Design your data discovery processes with compliance at their core, reducing the risk of legal issues.
6. Continuous Improvement and Adaptation
- Seek Feedback and Iterate: Regularly solicit feedback from users of your data discovery tools and processes and be prepared to make adjustments.
- Stay Informed About Technological Advancements: The field of data discovery is continually evolving. Stay informed about new tools and methodologies that can enhance your data discovery processes.
By implementing these strategies, organizations can significantly improve their unstructured data discovery processes, leading to better data management, enhanced security, and compliance with regulatory requirements. These practical steps provide a roadmap for tackling the challenges of unstructured data, turning a daunting task into a manageable and streamlined process.
Redefining Data Discovery with 1touch.io Inventa: A Tolly Group Benchmark
1touch.io Inventa, as recently benchmarked by the independent test lab The Tolly Group, has redefined the standards in data discovery for both structured and unstructured data. Employing advanced algorithms and machine learning techniques to ensure comprehensive and accurate data discovery, Inventa demonstrates exceptional accuracy and efficiency in diverse data environments. The Tolly report highlights its ability to navigate diverse data environments with industry-leading accuracy rates of 98.6% in structured data and 100% in unstructured data.
In benchmark tests, Inventa processed a database of 60 million rows in just 25 seconds and managed file shares with over 700,000 files efficiently, showcasing its capability in handling large-scale data at high speeds. This level of precision in data discovery and classification empowers organizations with comprehensive protection and efficient data management.
Navigating the Future of Data Discovery with Greater Precision
The accuracy of data discovery is foundational for robust data security, privacy maintenance, compliance adherence, and effective data governance. Inaccuracies in data discovery have far-reaching consequences, impacting not only operational aspects but also an organization’s legal standing and reputation.
Accurate unstructured data discovery is not just a technical necessity; it has become a strategic imperative. By employing effective strategies and utilizing advanced solutions like 1touch.io Inventa, organizations can navigate the complexities of unstructured data. This approach ensures enhanced security, compliance, and operational efficiency, positioning organizations for success in an increasingly data-centric landscape.
Download the Tolly Group Report for Transformative Data Discovery Insights
The Tolly Group report substantiates that 1touch.io’s platform is highly effective in discovering and classifying sensitive data, exhibiting not only high accuracy but also impressive throughput. Download the Tolly report today to discover how 1touch.io Inventa can transform your enterprise’s approach to sensitive data governance.
[/et_pb_text][/et_pb_column][/et_pb_row][/et_pb_section]