Managing the Risks of Generative AI: The Critical Role of Data Governance
Generative AI promises to transform businesses through enhanced efficiency, insights and customer engagement. Yet it also poses considerable risks around data privacy, security, ethics and responsible usage. Recent incidents have showcased generative AI’s downsides. With adoption accelerating rapidly, proactive data governance becomes essential to manage risks.
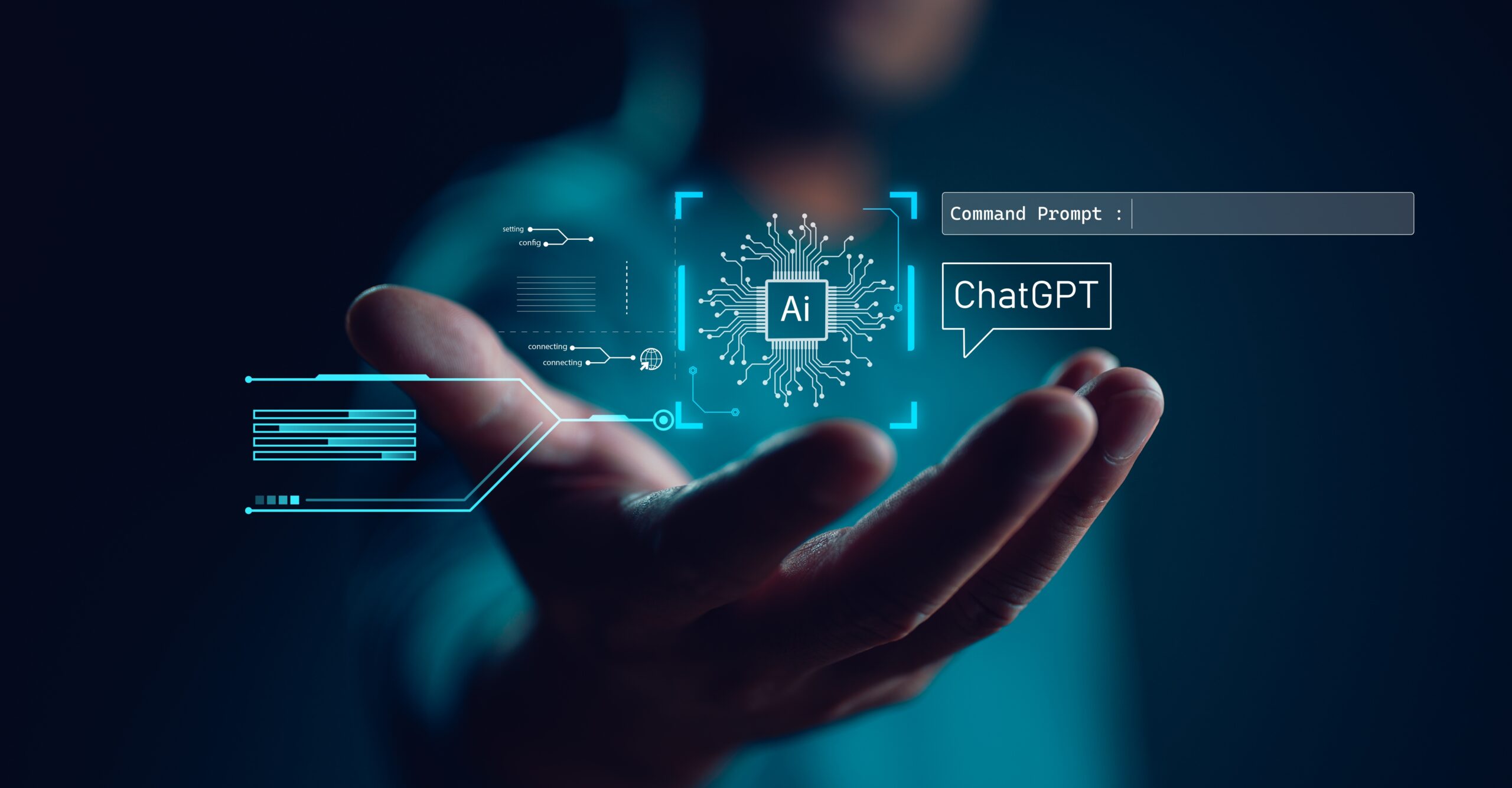
The Rise of Generative AI and Its Risk Profile
Generative AI refers to machine learning systems that can generate new, original content such as text, images, audio or video that reflects the data on which it’s trained. Prominent examples are chatbots like ChatGPT that can hold conversational dialog. But it also includes systems that generate images, 3D models, computer code and more based on textual prompts.
Key capabilities that enable generative AI systems include:
- Large neural network models with billions of parameters like GPT-4
- Massive training datasets scraped from the internet to emulate real-world complexity
- Reinforcement learning from human feedback
According to a recent IDC forecast, worldwide spending on generative AI is forecasted to reach nearly $16 billion in 2025, up from $300 million in 2020. [1] This exponential growth underscores how rapidly these technologies are spreading across organizations.
What’s driving this surge in interest? Generative AI promises to unlock insights, enhance workflows, and improve customer experiences across organizations.
Relevant use cases include:
- Marketing gains deeper insights from data to create targeted campaigns
- Customer support agents can quickly generate relevant responses to inquiries
- Recruiters source qualified candidates faster by generating job posts
- Legal teams perform contract reviews more efficiently
In a recent 1touch.io webinar, IDC analyst Jennifer Glenn stated:
“What digital transformation did for data, generative AI took a match and a room full of gasoline and just lit it on fire. Data is everywhere and people want to take advantage of it and harness it.” [2]
Indeed, generative AI brings data to life in new ways. But if mishandled, it could also burn organizations.
Key Risks Posed by Generative AI
While generative AI unlocks new opportunities, it also opens the door to significant risks if governance lags adoption. These dangers stem from both inherent model vulnerabilities and potential usage risks. Model risks involve threats of biases, errors, non-compliance, and harmful outputs, while usage risks relate to data loss, privacy infringement, and intellectual property theft.
Specific generative AI risks include:
- Data Privacy Violations: Models often train on massive public datasets, which may contain personal information. Inadvertent disclosure of such data violates privacy rights.
- Security Vulnerabilities: Exposing proprietary training data, commercial secrets or sensitive customer information through errors could lead to disastrous data exposure.
- Biases and Unfair Outputs: Since models learn from data, any biased decisions or language embedded in the training data gets amplified through the AI, leading to prejudiced outputs.
- Toxic or Dangerous Outputs: Models can potentially generate harmful content like hate speech, misinformation or violent imagery if not governed properly.
- Flawed Outputs and Hallucinations: Errors and nonsensical outputs remain a persistent issue with generative AI. In high-stakes scenarios like healthcare, such unreliable outputs can prove problematic.
- Difficult to Control: The black box nature of neural networks makes generative AI behavior difficult to predict or steer. Problematic outputs may emerge unexpectedly.
- IP Theft: Models could produce unauthorized content derived from copyrighted data or reveal proprietary algorithms, inventions or trade secrets.
- Model Risk: Performance drifts, emerging biases and blind spots in models over time require ongoing governance.
- Usage Risk: Individuals misusing models pose risks ranging from productivity decline to inappropriate content creation.
The risks arising from both technical and human factors underscore the critical need for governance over data flows, model training and usage. Otherwise, organizations are flying blind.
Real-World Incidents Reveal Generative AI Dangers
Several recent incidents have provided sobering examples of how generative AI can backfire if governance is lacking:
- Microsoft Data Exposure: Researchers published open source AI training data, exposing 38TB of sensitive corporate data including employee backups, passwords, secrets and Teams chat messages. [3]
- Extracting ‘Deleted’ Data: Scientists from the UNC Chapel Hill showed sensitive could still be extracted from models like ChatGPT, even after deletion, raising compliance and privacy concerns for training data. [4]
- Lack of Ethical Guidelines: A Deloitte Technology Trust Ethics report found 56% of professionals were unsure if their company has generative AI ethical guidelines, even as 74% actively test the technology. [5]
- Data Privacy Worries: In the same survey, 22% of respondents cited data privacy as their top concern about generative AI, while 14% worried about training data transparency. [6]
These examples provide a reality check: deploying generative AI without governance invites risks.
The Generative AI Data Governance Gap
How prepared are companies to govern generative AI data and usage? An IDC survey reveals a concerning gap:
- Only 30% have proactive, enforced data governance policies for generative AI.
- 26% have published guidelines but don’t enforce them fully.
- 26% have provided limited, informal guidance. [7]
Many organizations recognize the advantages of generative AI but aren’t putting proper governance guardrails in place. This dilemma of maximizing potential while minimizing risk raises significant concerns about data security, privacy, and governance. Depending solely on humans to govern generative AI data and models at scale is unrealistic. Yet legacy tools also fall short.
Traditional Tools Fall Short in Managing Generative AI Risks
Most organizations rely on traditional data security tools like classification, encryption and data loss prevention (DLP). While helpful, these solutions alone are inadequate for governing complex generative AI for several reasons:
- They lack real-time, nuanced capabilities required for continuously evolving AI systems where the logic and decision-making process is difficult to interpret or audit.
- Generative AI integrates vast unstructured data sets like documents and communications across diverse repositories, posing discovery, classification and oversight challenges.
Governing and managing generative AI risks poses major challenges:
- Lack of Clear Governance Frameworks: Requiring internal guidelines tailored to each organization’s specific needs.
- Need for Granular Data Visibility: Gaining comprehensive visibility into distributed data is difficult but essential for managing sensitive information.
- Unstructured Data Complexities: Massive volumes of unstructured data make classification and management challenging.
- Validating Synthetic Data: Verifying accuracy and origin of synthetic training data is crucial yet difficult.
- Coordinating Across Teams: Different groups involved require tailored data governance strategies.
Overcoming these hurdles necessitates thinking beyond legacy tools to create an integrated, scalable approach customized to generative AI’s evolving risks.
A Proactive Approach to Data Governance for Generative AI
To manage risks responsibly, data governance needs to be built into generative AI systems from the initial design stage.
Key data governance elements should include:
Governance Strategy
- Develop internal data governance policies tailored to your organization’s specific generative AI use cases.
- Appoint dedicated data stewards spanning security, privacy, compliance and responsible AI to oversee governance processes.
- Maintain human oversight of ethics, fairness and societal impacts and implement approval gates before deploying applications.
Tools and Technology
- Implement AI data governance platforms providing end-to-end sensitive data discovery, automated classification, policy enforcement and analytics.
- Architect solutions integrating security and compliance via APIs, microservices and connections to data catalogs, data lakes, and other repositories.
- Prioritize scalable platforms leveraging machine learning to govern exponential data growth.
Training and Communication
- Mandate training on responsible data, security protocols, and governance policies specific to generative AI.
- Promote transparency by documenting data flows, model logic, and oversight procedures to build trust.
Risk Assessments
- Continuously evaluate risks related to data privacy, model biases, unfair outputs, security vulnerabilities, integration points and regulatory non-compliance.
- Perform rigorous impact analysis and risk assessments before launching any new generative AI project.
Monitoring and Testing
- Actively monitor models post-deployment for performance drifts, emerging biases, and aberrant behavior.
- Continuously test for and mitigate risks like data quality issues, model biases, and unfair outputs.
- Conduct ongoing audits to catch compliance policy drifts or violations early.
Human Judgment
- Ensure generative AI oversight teams have cross-disciplinary expertise in data science, security, ethics and governance.
- Enable human judgment calls on data ethics, model fairness, and performance issues to complement technical governance.
Adopt an AI TRiSM Approach
Gartner has highlighted AI Trust, Risk, and Security Management (AI TRiSM) as the number one strategic technology trend for 2024.[8] AI TRiSM provides a framework to build protections into AI systems and establish robust AI governance. Elements of an AI TRiSM program align with the data governance imperatives outlined here, including privacy, security, explainability, and continuous model monitoring.
Gartner predicts that by 2026, enterprises employing TRiSM controls will increase decision-making accuracy by eliminating 80% of faulty generative AI outputs. With a comprehensive governance strategy incorporating leading practices like AI TRiSM, organizations can securely harness generative AI and minimize blind spots.
Conclusion: Data Governance is Key to Managing Generative AI Risks
As generative AI adoption accelerates, overlooking governance invites unnecessary risks ranging from non-compliance to reputational damage. By taking a proactive approach, organizations can benefit from generative AI securely and responsibly. The imperative now for security, privacy and compliance leaders is to recognize AI data governance gaps. With proper governance, companies can strategically tap into generative AI, harnessing data’s full potential while upholding ethics and protecting sensitive information. The principles of automation, integration, transparency, and human oversight serve as guideposts to realize generative AI’s promise while controlling the risks.
Take Control of Your AI Data with 1touch.io Inventa
Navigating the complexities of Generative AI can be daunting, but AI-powered solutions like 1touch.io Inventa simplify the complexities of generative AI. Inventa offers sensitive data intelligence, enabling organizations to safely leverage their entire data estate, including structured and unstructured data, for AI and analytics projects. By maintaining robust security controls and providing comprehensive visibility into data sensitivity and classification, it enhances time to insight while protecting sensitive information.
Optimize Generative AI with Precision and Protection
Don’t let data governance gaps hold your organization back from harnessing generative AI securely. Get our white paper, 1touch.io Inventa: Optimize Generative AI with Precision and Protection, for insights and guidance on implementing robust data governance for AI. With the right data governance strategy tailored to your unique use cases, you can confidently innovate with AI and protect what matters most.