How AI is Transforming Data Governance in 2023
Data has become one of the most valuable assets for modern enterprises. Yet, as organizations amass more data across systems, governing and safeguarding this information grows increasingly challenging. Traditional governance methods—characterized by manual monitoring, siloed policies, and reactive protocols—are ill-equipped to cope with the burgeoning volume of data, the diversity of users, the breadth of applications, and the spectrum of threats.
Artificial Intelligence (AI) offers significant enhancements to data governance. As we wrote previously in “The Impact of AI and Machine Learning in Data Security,” the integration of AI and ML technologies into the enterprise has transformed data security. This transformation is evident in sophisticated threat detection, real-time monitoring, and enhanced efficiency and scalability. Similar transformative impacts can be realized when AI is applied to data governance.
By integrating AI into data governance programs, essential insights are connected across users, policies, risks and systems. Advanced automation, real-time adaptation, and scalable analysis of massive datasets bolster data governance strategies, elevating them to the next level.
Today’s post examines the limitations of traditional data governance, the enhancements AI offers, and the key considerations for implementing AI into your data governance practice.
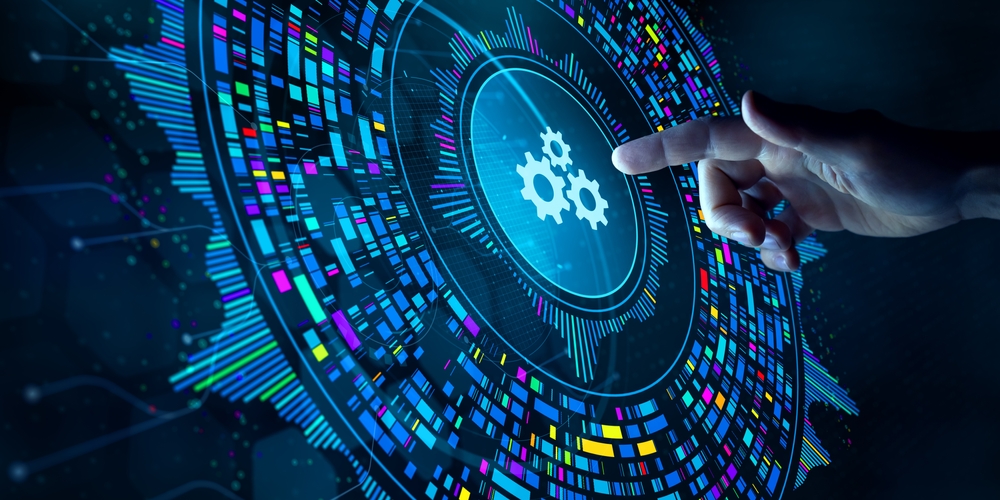
The Limitations of Traditional Data Governance Approaches
Data governance involves managing data availability, integrity, security, and usability within an organization through policies, procedures, standards, roles, and metrics. Acceleration Economy succinctly explains, governance is “where risk management meets ethical standards.”
Effective governance is pivotal for mitigating risk, maintaining compliance, and maximizing data’s value. The rapid growth in generative AI across enterprises only serves to underscore its essential role in overseeing expanding data ecosystems. An article by CIO emphasizes, “Rapid acceleration in artificial intelligence, particularly generative AI are putting more pressure on analytics and IT leaders to get their houses in order when it comes to data strategy and data management.”
A recent Salesforce report found that “87% of technical leaders say that advances in AI make data management a higher priority and 92% say that trustworthy data is needed more than ever before. IT leaders say that the requirements for successful gen AI use include accurate, complete and unified data (55%), enhanced security measures to avert new threats to the business (54%), and ethical use guidelines (30%).”
However, traditional governance practices struggle to keep pace with the modern data landscape, constrained by unscalable manual processes, fragmented policies, reactive protocols, and limited visibility. These deficiencies often result in undetected policy violations, an inability to trace data lineage, insufficient insights for decision-making, and regulatory non-compliance.
Common pitfalls include:
- Manual Processes Unable to Scale: Data mapping, policy enforcement and threat monitoring still rely heavily on human effort, creating gaps as data growth outstrips resources.
- Fragmented, Inconsistent Policies Across Silos: Governance frameworks often differ and are inconsistent across units, heightening risk and complicating data usage.
- Reactive Threat Response: Issues get addressed post-incident, instead of preventing threats proactively, often when the damage has already been done.
- Limited Visibility with Growing Data Volumes: Achieving unified visibility into governance health is nearly impossible to achieve manually with so many systems and repositories.
- Inflexible Policies Misaligned with Real-World Usage: Traditional top-down governance doesn’t tend to reflect how people actually interact with data.
These shortcomings can lead to unchecked policy violations, blind spots in data lineage, lack of decision-making insights, and non-compliance with expanding regulations. According to CIO, “94% of technical leaders say they should be getting more value from their data and 78% say their organizations struggle to drive business priorities with data.” Indeed, “increased data volume and complexity also exacerbates the difficulty of data harmonization, making it difficult to extract value from data sources.”
Today’s complex data landscape demands updated governance strategies. Security and risk leaders need to modernize governance strategies to control the volumes of data their organizations rely on. AI is invaluable in this context.
Eight Ways AI is Transforming Data Governance
As demonstrated in the realm of security, AI and ML technologies hold the promise of transforming data governance by automating manual tasks, providing real-time visibility and adaptability, and handling extensive datasets.
Applied to sensitive data governance, AI-powered processes unlock new possibilities:
- Automated Policy Monitoring and Access Control: Manually monitoring user access and activity to ensure policy adherence is no longer feasible given data volumes and velocity. AI algorithms can continuously monitor audit trail activities, detect anomalies, and restrict access automatically based on policy rules, strengthening enforcement and freeing up human resources.
- Accelerate Data Mapping and Lineage Tracing: For governance, understanding data connections and sources of data elements is critical, but manual mapping for large enterprises is time-consuming. AI instantly traces data flows, maps dependencies, flags sensitive data, and identifies policy gaps.
- Proactive Regulatory Compliance and Controls: AI models trained to understand regulations can autonomously scan data, categorize it based on rules, restrict non-compliant usage, and recommend remediation steps, transitioning compliance from a reactive to proactive stance.
- Monitor for Anomalies and Threats in Real-Time: Manual identification of threats across data environments is challenging. AI’s ability to monitor continuously, spot patterns, and predict risks offer a formidable defense.
- Utilize Predictive Analytics for Governance Planning: AI algorithms forecast governance risks by analyzing datasets on user behavior, access patterns, and past incidents, enabling preemptive policy changes and controls.
- Manage Metadata and Data Cataloging: Cataloging data manually for governance in massive unstructured datasets is nearly impossible. AI rapidly scans datasets, extracts metadata, classifies data types, and auto-tags them for easy discovery and access management.
- Empower Business Teams with Data Insights: AI can accelerate data democratization by providing business teams with instant insights for decision making while governing access.
- Scale Governance with Organizational Growth: As data landscapes grow, scaling governance is a priority. AI’s scalability supports increased volumes without sacrificing accuracy or oversight, ensuring policies grow with the company.
With vast data growth across on-premises and cloud resources, the value of AI-driven governance is tangible and substantial, providing operational efficiencies, cost reductions, and strategic advantages. Enterprises are witnessing a considerable uptick in the efficiency and effectiveness of governance functions, attributing this success to AI integration.
Implementing AI in Data Governance: Considerations for Success
Integrating artificial intelligence into data governance practices offers a robust solution to the complexities of managing voluminous data. AI’s role in data governance streamlines operations, maintains compliance, and strengthens security, allowing for strategic data utilization.
As we’ve explored in our previous article, risk assessments, data governance, monitoring, and human oversight are necessary to responsibly deploy AI in security. These foundational measures also underpin the ethical and effective governance of data through AI.
Here’s how enterprises can start leveraging AI to elevate their data governance efforts:
- Identify Strategic Areas for AI Implementation: Start by identifying data governance areas where AI can have the most impact, such as automating compliance checks, optimizing data quality management, or enhancing data security and privacy.
- Implement a Pilot Program: Launch a focused pilot program targeting a specific governance issue before expanding AI usage. This approach allows you to demonstrate the value of AI in a controlled setting and refine your strategy based on tangible outcomes.
- Ensure Data Quality: AI systems demand high-quality training data to function optimally. Dedicate time and resources to verify your data’s accuracy, completeness, and representativeness to avoid biases and ensure reliable AI insights.
- Provide Training and Support: Equip your data governance team with the necessary training and resources to effectively utilize AI tools. This includes understanding AI capabilities, interpreting results, and weaving AI insights into governance frameworks.
- Maintain Human Oversight: AI can automate many aspects of data governance, but human expertise remains crucial. Implement a system of checks and balances where data governance professionals supervise AI operations to ensure that policies are applied correctly, and compliance is achieved.
- Adopt Transparent AI Practices: Develop AI systems with transparency at their core. Ensure there is comprehensive documentation on how the AI operates, its decision-making process, and the actions it performs, fostering trust and understanding among all stakeholders.
- Monitor and Adjust Continuously: AI in data governance is not a set-it-and-forget-it solution. Continuous monitoring and periodic adjustments are required to adapt to new data sources, evolving regulations, and emerging threats.
- Integrate with Overall Data Strategy: AI-driven data governance should be an integral part of a broader data management and analytics strategy, aligning with the organization’s objectives and encouraging cross-functional collaboration.
By adopting these strategic measures, organizations can harness the power of AI to significantly upgrade their data governance, achieving efficiency levels, risk management, and strategic insights.
Embracing the Future: AI’s Critical Impact on Data Governance
AI is redefining the foundation of data governance in 2023, delivering advanced capabilities in automation, predictive analytics, real-time threat detection, and scalability. As enterprises increasingly recognize the limitations of traditional governance methods, the move toward AI-enhanced data governance systems is gaining momentum. These systems are not only optimizing operations but are also ensuring that enterprises stay responsive and compliant within the fast-changing data landscape. It’s time for enterprises to look beyond the conventional and embrace the transformative potential of AI for data governance.
Implementing AI-Driven Data Governance with 1touch.io
1touch.io Inventa harnesses the power of AI to modernize data governance, addressing the very challenges outlined above. It is not just about replacing outdated systems; it’s about enhancing data governance frameworks with intelligent automation, real-time analysis, and predictive insights—transforming reactive governance into a proactive stronghold.
By integrating 1touch.io Inventa into your data governance strategy, your enterprise is not just keeping pace with technology—it’s setting the pace. It’s a strategic move towards a more secure, compliant, and efficient future.